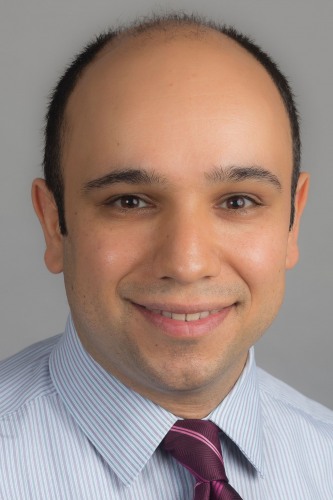
Amin Zand Vakili, MD, PhD
Awards
Advance-CTR Mentored Research Awards (2018)
"Machine Learning to Predict Clinical Response to Transcranial Magnetic Stimulation: A Resting State Electroencephalography Study"
Major Depressive Disorder (MDD) is a common and debilitating illness. It affects a person's family and personal relationships, work, education and life. It changes sleeping and eating habits and significantly impairs patients’ general health. The disorder affects Veterans more than the general population, both as an isolated illness and in conjunction with posttraumatic stress disorder (PTSD) and suicidality. Symptoms in a notable proportion of patients (~30%) do not respond to behavioral and pharmacological interventions and new treatments are in great need. One such treatment, transcranial magnetic stimulation (TMS), has been cleared by Food and Drug Administration for treatment in MDD. TMS is effective in around 60% of patients with treatment-resistant MDD but is associated with significant financial and time burden. Further insights into the neurobiological effects of TMS and markers for functional recovery prediction and treatment progression are of great value. The goal of this proposal is to use human electrophysiology (electroencephalography, hereafter EEG, in particular) and machine learning to predict treatment response in candidates for TMS treatment and also study TMS’s mechanism of action. Doing so has several benefits for patients, as prediction of treatment helps providers in screening out the patients for whom TMS is ineffective and understanding the mechanism allows us to refine and individualize the treatment. We will recruit 35 patients with treatment-resistant MDD and record resting state EEG signal with a dense electrode array before and after a 6-week clinical course of TMS treatment. We will use machine learning (Sparse regressions) to predict treatment outcome using functional connectivity (Coherence) maps derived from EEG signal. We also will use classifiers to track changes in functional connectivity through the course of treatment. Based on our preliminary data, we hypothesize that weaker functional connectivity between prefrontal cortex (where the stimulation is delivered) and parietal/posterior midline sites predict better response to treatment and that TMS treatment will enhance these connections. The data collected here would be used as a seed and preliminary data for future federal (NIH and the VA) career development awards which will focus on use of EEG to better understand brain function and neuromodulation treatments.