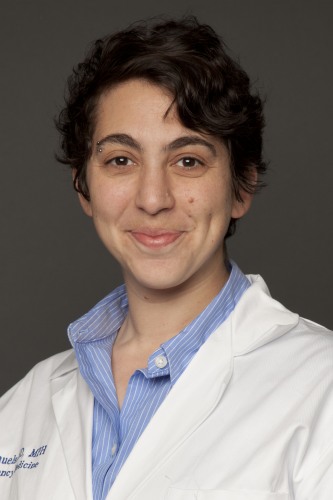
Elizabeth Samuels, MD, MPH, MHS
Awards
Advance-CTR Mentored Research Awards (2019)
"Identifying Opioid Overdose Hotspots for Prevention and Treatment Resource Deployment"
In the last decade, opioid overdose has become a leading cause of injury and death in the United States. Rhode Island (RI) has one of the highest rates of opioid overdose death and has been a national leader in state-wide policy innovations to address the epidemic. Most overdose prevention and treatment strategies have been implemented on a state-wide basis and there remain gaps in access to services to prevent overdose death and addiction treatment. Knowing where and when overdoses occur would enable targeted resource allocation in communities most impacted by the opioid overdose epidemic. However, RI’s current surveillance mechanisms to identify clusters of opioid overdose cases, or hotspots, are delayed and lack the geographic precision necessary to enable targeted community interventions. Over the last four years, Dr. Samuels has worked with RI emergency departments and the RI Department of Health (RIDOH) to develop and implement emergency department policies for post-overdose care and linkage to addiction treatment. Through this mentored training award, Dr. Samuels will build on this foundation to identify opioid overdose prevention and treatment services within opioid overdose hotspots and identify neighborhood factors associated with opioid overdose. These findings will be used to inform targeted community interventions to reduce opioid overdose and improve treatment engagement.
Big Data Pilot Project Awards (2017)
"ED SMART NOTIFICATIONS: REAL-TIME PREDICTIVE MODELING OF AT-RISK EMERGENCY DEPARTMENT PATIENTS"
CO-PIS: MEGAN RANNEY, MD, MPH (CONTACT PI), FRANCESCA BEAUDOIN, MD, PHD, and BRANDON MARSHALL, PHD
Frequent ED visits serve as a marker of multiple health risks. Although public health and governmental bodies call for identification of these at-risk patients and linkage to treatment, current systems make it difficult to identify patients at risk of frequent ED visits (and related comorbidities) at the time of an ED visit. This pilot study will use existing big data from statewide health records to develop preliminary predictive analytic models, which will later be implemented in real-time during ED visits to help improve this at-risk population's health status.