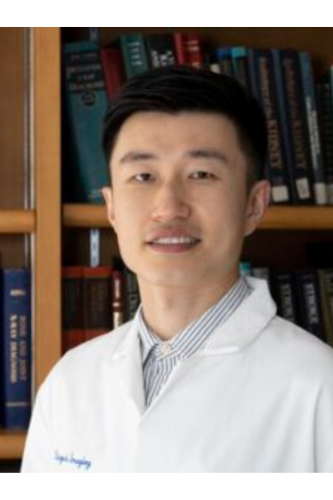
Zhicheng Jiao, PhD
Research
Advance RI-CTR Pilot Projects Program (Cycle 7)
"Generalizable Artificial Intelligence for Large-Scale Privacy-reserved Covid-19 Study"
The burst of positive COVID-19 cases drastically increases the workload of radiologists, making it
challenging to keep the burden of patients within the treatment capacity of the local healthcare system. The proposed research uses artificial intelligence (AI) methods based on longitudinal chest x-ray images and clinical data to predict the clinical outcome of COVID-19 patients by building a large-scale multi-institutional dataset and developing a privacy-preserved AI pipeline, and we will demonstrate the generalizability and broad applicability of our algorithm in a large-scale multi-institution setting. Our models have the potential to assist in personalized treatment planning and timely resource allocation while relieving the workload of radiologists and providing references for other emergency public health issues.
Our long-term objective is to develop and deploy generalizable privacy-preserved artificial intelligence (AI) pipeline for clinical outcome prediction in COVID-19 patients, aiming to improve personalized treatment planning and timely resource allocation while relieving the workload of physicians providing references for other public health emergencies. The feasibility of chest x-ray makes it widely used in providing huge assistance to clinicians for screening, diagnosis, and prognosis of COVID-19 patients. As a result, radiologists need to spend way more time reading these images to detect the lesion areas and further compare several longitudinal chest x-rays to seek more progression-related findings. The increasing number of chest x-ray images will drastically increase the workload of radiologists, especially when there is a burst of positive cases such as the Delta and Omicron variants, which have been leading to an overwhelming increase in hospitalizations in some states.
In recent studies, we have demonstrated that AI models based on the combination of imaging and clinical data perform well in diagnosis and prognosis. However, the current models proposed in our papers are formulated in a 2-stage fashion that separately learns feature representation for evaluating severity and links these features with the clinical outcomes of progression to critical disease, which may limit prediction accuracy without utilizing the end-to-end learning from big data ability of deep neural networks. Besides, the limitation of existing models optimized on single-cohort data causes decreased prediction performance on external testing sets, making the developed AI models less usable when deploying them on other institutions.
The proposed research aims to develop novel AI architectures based on routine chest x-rays and clinical data that allow for generalized and privacy-preserved prediction of clinical outcomes in COVID-19. Using large scale data from Rhode Island Hospital and our collaborated institutions, our project will
(1) develop end-to-end deep learning AI models to learn efficient feature representation from both imaging and clinical data and link them with COVID-19 outcomes to achieve improved clinical outcome prediction accuracy;
(2) deploy more generalizable AI models via participating in a multi -institution collaboration to organize a large-scale, most heterogeneous COVID-19 chest x-ray dataset ever;
(3) achieve privacy-preserved outcome prediction of COVID-19 patients from multi-site data via a multimodal federated learning pipeline. In addition, we will compare results with those of radiologists and combine manual and AI prediction for a complimentary analysis. The proposed research will significantly advance the translation and clinical adoption of AI-based COVID-19 clinical outcome analysis in a large-scale and multi-institution setting, providing assistance for fighting the pandemic via improving community health resources and service, and references for other public health emergencies.