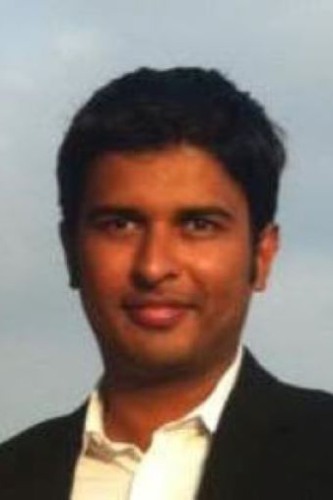
Adeel Abbasi, MD, ScM
Awards
Advance-CTR Pilot Projects Program (2020)
"Predicting Hemorrhage and Thrombosis in Mechanical Circulatory Support: A Study Using Machine Learning"
Mechanical circulatory support (MCS), which includes cardiopulmonary bypass (CPB) and extracorporeal life support (ECLS), has been widely adopted but severe hemorrhage and thrombosis remain significant causes of morbidity and mortality. Very little is understood about predictors of these devastating complications, which is likely influenced by a multitude of complex patient and clinical factors, and their innumerable and dynamic interactions over time. Standard methodologic approaches to understand these interrelationships have proven unsuccessful. Some of these factors are modifiable and if discovered poor outcomes in MCS may be preventable. The long term goal of the PI’s research is to prevent poor outcomes in MCS by using machine learning, a robust artificial intelligence methodology ideally suited but yet to be adequately leveraged in MCS. The PI’s central hypothesis is that machine learning applied to varied MCS datasets will identify key and as yet unknown modifiable predictors of hemorrhage and thrombosis. Three datasets that capture the use of CPB and ECLS in retrospective patient cohorts will be created or leveraged: patients supported with 1) ECLS at Rhode Island Hospital, 2) ECLS within the Extracorporeal Life Support Organization (ELSO) registry and 3) CPB within the Society of Thoracic Surgeons (STS) Adult Cardiac Surgery Database (ACSD). The study hypothesis will be tested by generating machine learning models to predict hemorrhage and thrombosis in these datasets, and cross-validated using validation cohorts within the larger ELSO and STS databases.